

Participants are recruited into the study over a period of two years and are followed for up to 10 years. These issues are illustrated in the following examples.Ī small prospective study is run and follows ten participants for the development of myocardial infarction (MI, or heart attack) over a period of 10 years. In the first instance, the participants observed time is less than the length of the follow-up and in the second, the participant's observed time is equal to the length of the follow-up period.
Regression exp(b) spss 23 free#
This can occur when a participant drops out before the study ends or when a participant is event free at the end of the observation period. The most common is called right censoring and occurs when a participant does not have the event of interest during the study and thus their last observed follow-up time is less than their time to event. There are several different types of censoring. What we know is that the participants survival time is greater than their last observed follow-up time. True survival time (sometimes called failure time) is not known because the study ends or because a participant drops out of the study before experiencing the event. In each of these instances, we have incomplete follow-up information. Some participants may drop out of the study before the end of the follow-up period (e.g., move away, become disinterested) and others may die during the follow-up period (assuming the outcome of interest is not death). Thus, participants who enroll later are followed for a shorter period than participants who enroll early. In many studies, participants are enrolled over a period of time (months or years) and the study ends on a specific calendar date.
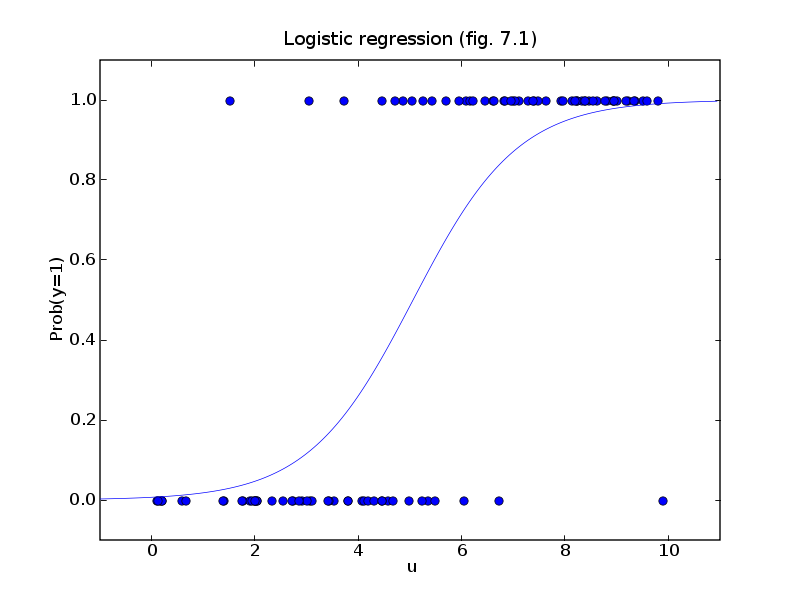
Specifically, complete data (actual time to event data) is not always available on each participant in a study. Nonparametric procedures could be invoked except for the fact that there are additional issues. Standard statistical procedures that assume normality of distributions do not apply. On the other hand, in a study of time to death in a community based sample, the majority of events (deaths) may occur later in the follow up. For example, in a study assessing time to relapse in high risk patients, the majority of events (relapses) may occur early in the follow up with very few occurring later. First, times to event are always positive and their distributions are often skewed. There are unique features of time to event variables.
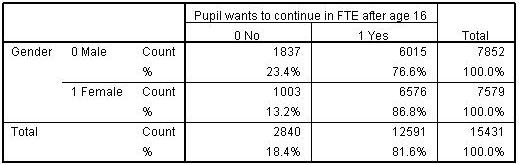
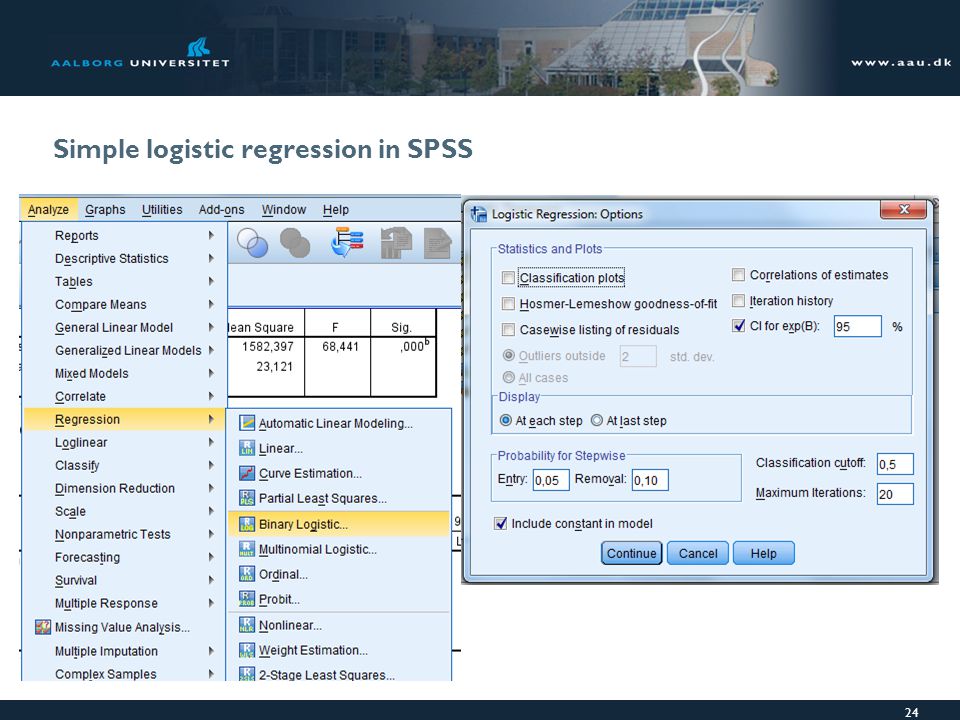
